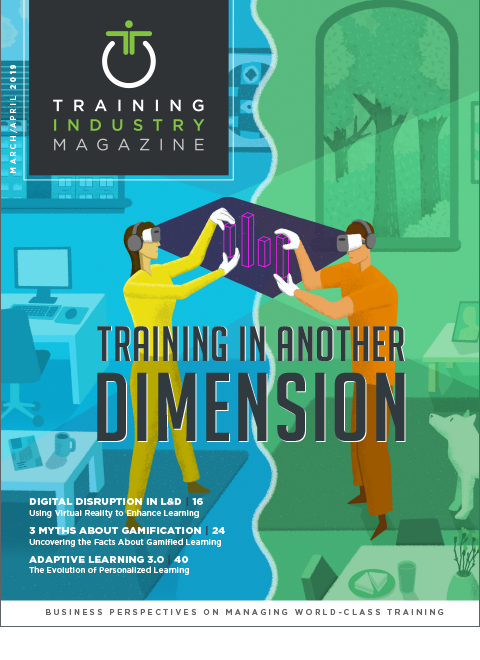
Published in Mar/Apr 2019
Artificial intelligence and machine learning continue to take on a greater role in the learning and development field: providing personalized learning paths, predicting our performance, and making recommendations on content and activities. This year, AI systems will support our workplace learning trajectory more than ever, but only if we can be deliberate in establishing ethical frameworks and make its use more transparent. Below are some of the challenges we face as we develop AI applications for learning and some potential ways to address ethical concerns.
Current Challenges
- Black box algorithms: Increasingly, researchers are saying that algorithms used by organizations can be opaque and discriminatory. Black box algorithms refer to the general inability to see inside of a system and see how it arrives at a decision. For example, when a learning platform makes recommendations to a learner, how would you know what learner attributes the platform uses as criteria for such recommendations and if they are conducive for learning. Even more troublesome, there are few options, unless you are a computer programmer, for updating and tweaking these assumptions and bias that are inevitable in these systems.
- Issue of trust: In measuring learning and understanding how and what to personalize to individual learners, companies and platforms are collecting a huge amount of personal data. At the same time, ownership and governance of data is often ill-defined, or not defined at all, for many organizations. Lack of definition promotes a feeling of mistrust among employees, while data is being collected without clear and conscious learner consent. In today’s “big brother” surveillance culture, people don’t inherently trust machine learning algorithms, especially when the decision-making process behind the scene is lacking transparency and accountability.
- Lack of guiding principles and governance: While tech giants such as Google, Microsoft , and SAP have all introduced ethical principles for AI, there is a lot of room for more concrete definitions and better oversight. One criticism over AI principles set by tech firms is that they are more concerned with protecting themselves from legal actions and less on coming up with a realistic, actionable set of guidelines. In the L&D world, the issue is further complicated by changing standards in e-learning and learning management system technology whereby learner profiling and data tracking are often not made explicit and without user consent.
Potential Resolutions
- Explainable AI: One notion to counter black box algorithms is advocating the use of explainable AI, XAI. Essentially, XAI programs enable users to understand the technology, provide input on the decision-making process and improve algorithmic accountability. In the case of dynamically generated learning paths, learning platforms should be explicit about the decisions it makes to recommend certain learning paths or options over others. In addition, learners, course designers, and instructors are given the ability to review and update as they see fit.
- Privacy by design: Privacy needs to be considered by the whole design process, not just as a bolt-on approach. Privacy by design is a system engineering approach proposed by Dr. Ann Cavoukia. Essentially, privacy by design advocates that organizations need to consider privacy at the initial design stages and throughout the development process of new products or services that involve handling personal data.
- Regulatory frameworks for AI: Regulatory frameworks are needed to safeguard transparent and auditable education data. Prejudices of the past must not be unwittingly built into AI-enabled learning systems. Systems and algorithms need to be carefully designed, explained, and audited. While government plays a key role in setting regulatory frameworks, organizations should collaborate and encourage discussions on the ethics of AI by setting up advisory bodies, developing core ethical principles and responsibly handling data.
For AI to deliver its promise on learning enhancement, it will require people to trust the technology, organizations to safeguard the use of personal and educational data, and for government to collaborate with industries to research and discuss ethics in AI.